admin | 18/02/2020
Being able to predict failures and improve process productivity are the goals of every industry, and they are getting closer to being achieved thanks to the implementation of new technology. In the last few years, digital twin technology has played an outsize role in this endeavor. The technology can demonstrate the impact of design changes, application scenarios, the incorporation of new components, and many other circumstances virtually, without having to invest in physical assets.
According to Pablo Ayala, the coordinator of Visual Intelligence Technology at GAIA (Association of Electronic and Information Technologies in the Basque Country), digital twins are systems that reproduce or represent critical components of production systems virtually so as to improve their efficiency. In order to create these highly precise representations, multiphysics, data analysis, artificial intelligence, machine learning, and three-dimensional visualization functionalities are used.
There are three types of digital twins, depending on their application:
– Product. These digital twins allow product performance to be validated virtually, and they show how the product will work in the real world before it is manufactured. This reduces costs arising from experimenting with new materials or designs without costly prototypes, improving the quality of the finished product and simulating many more stress conditions than possible in a laboratory.
– Production processes. By creating a digital twin and analyzing its efficiency in different conditions, researchers can save time vis-à-vis idle equipment and improved task scheduling, including tasks such as preventive maintenance.
– Performance. These work to collect and analyze data on the industry database, leveraging all of the information from the plants in operation to make better decisions.
“The main benefit is efficiency, both with regard to product and process. Having these twins allows us to understand and predict the asset’s performance, processes, and products; foresee situations of potential misuse, failures, and gaps; and identify improvements in efficiency,” affirms Ayala.
A market on the rise
The companies that supply this software use a myriad of technologies in the process of modeling their products and production chain: data from sensors installed in physical objects to determine real time performance and changes; digitalized engineering and design plans; and information from third parties, such as the manufacturers of the machinery being used. They can even use the weather if it affects the facility (as can happen in outdoor operations, for example). “All of this Big Data is combined and becomes interrelated, creating such strong links that the data provide feedback to other data until an evolving environment is created — one that adapts to the different circumstances and modifications that come up in real time during factory operations,” says GAIA’s Visual Intelligence Technology coordinator.
The digital twin market is expected to grow from 3.8 billion dollars in 2019 to 35.8 billion in 2025, with a compound annual growth rate of 45.4%, according to the consultancy Markets and Markets. The factors that are driving demand for digital twins include the growing incorporation of emerging technologies such as IoT and the cloud – in their implementation – as well as the promising outlook of their incorporation into industries including aerospace, defense, automotive, transport, and healthcare.
Digital twins are systems that are critical components for production systems to be able to improve efficiency and predict failures
One of the most significant characteristics of digital twins is that they are adaptable to all kinds of sectors and industrial environments, because they are aimed at improving the product or the process. In fact, “thanks to their application, industries can demonstrate the impact of design changes, use cases, environmental conditions, and an extensive array of other variables.” Ayala offers up the example of a company that wants to simulate how its assembly line would work if it added more people to one area or incorporated a robotic arm or an AVG to transport materials. “This way, improved cycle times can be verified before making an investment or actually modifying the line,” he claims. This is a situation that can be reproduced for processes in oil and gas refineries, traffic networks, wind farms, hospitals, etc.
Specifically, the auto and transport industries will be key for digital twins in the near future, thanks to their use in design, simulation, maintenance, repair and review, production, and subsequent service. These industries are also pioneering the adoption of these technologies, and there is a growing demand for connected devices in these sectors due to advances such as connected vehicles and self-driving vehicles, which, in turn, drive the growth of the market.
Technological adaptation
The use of virtual representation systems is not new, as the GAIA expert admits, but the widespread application of 4.0 standards in the industry has entailed a great deal of interest in and development of these digital systems, which reproduce or represent critical elements in the efficiency of production systems. So much so that a study by the consulting firm Gartner forecasts that by 2021 approximately half of all industrial companies will use digital twins, allowing for a 10% increase in global efficiency.
This interest has been reflected in a push for the implementation of these kinds of solutions from public entities around the world. That has developed into a number of training and outreach measures, “creating a very positive ecosystem for industrial firms, which have a great deal of interest in developing, automating, and streamlining.” The advantage here is that it’s not only large companies whose interest has been piqued, but also that of small and medium enterprises. There are also programs and frameworks that support, on the one hand, the design of these kinds of solutions and, on the other, their implementation within the industrial sector.
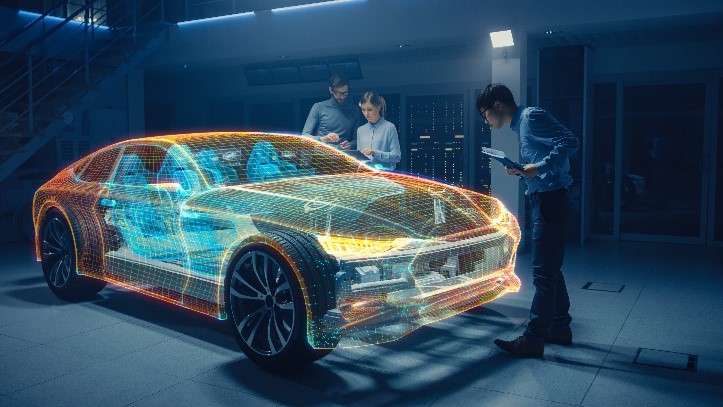
The use of digital twins has a promising outlook in industries such as aerospace, defense, auto, transport, and healthcare.
“The most important task will be highlighting the great deal of potential that digital twin technology has in the industry with success stories demonstrating the return on investment and the numerous advantages it offers,” underscores Ayala. The companies that develop these solutions will therefore have to transform their know-how into new services and solutions specific to the industrial sector, and, above all, be able to do it quickly and in a streamlined fashion.
Designing the application of digital twins requires a great deal of technical expertise on their entire process — “in other words, organizational maturity.” These organizations must fully transform their structures. To do this, they need to implement the following:
– Monitor and digitize processes requiring strict integration architecture and industrial automation on a massive scale.
– Expand the capability of current data storage, management, and analysis systems to handle the large amounts of data required by digital twins.
– Go from using digital representations to multiple simultaneous digital copies with greater ability to assess alternative scenarios.
Ayala, therefore, considers the technology to be accessible; but in order to be able to develop all of its potential, in addition to having the necessary internal resources, companies must commit to planning the processes and getting the most out of this technology. “Given the growing trend of digitization in all sectors, limits cannot be put on the use of these new virtual environments,” he states.
How is a digital twin made?
Uses of digital twins
The application scenarios for digital twins notably include:
- Checking whether a product is in compliance with the specifications devised in the design before making a real prototype or starting production.
- Identifying whether a product is stable and intuitive without having to pay for costly laboratory tests or large-scale testing.
- Monitoring potential product failures based on many more simulations than would be possible in a traditional environment.
- Detecting inefficiencies or areas for improvement within an industrial plant’s manufacturing processes.
- Improving preventive maintenance of the machinery being used and testing different production models without having to implement them into everyday operations, thus reducing the risks associated with these changes.
- Reducing the amount of idle time in factories and optimizing energy consumption.
- Creating a single viewing point for data from factories, centralizing the company’s Big Data, reducing administrative costs, and providing better input for directors to be used in managing the business.
Article collaborator…
Pablo Ayala is a computer engineer and serial entrepreneur. In the last 15 years, he has worked to launch a number of businesses and companies related to innovative technologies. He is currently the CEO of Innovae (www.innovae.eu), an Industry 4.0 augmented reality and virtual reality company.
He is a member of the Board of Directors of GAIA, the Association of Electronic and Information Technologies in the Basque Country (www.gaia.es), and coordinator of Visual Technology.
He works with several training centers and universities as a professor in master’s programs on Industry 4.0 and Smart Manufacturing. With his supportive and entrepreneurial spirit, he is the co-founder of Berriup, a start-up accelerator, and Eywa Space, a venture builder, specializing in the area of extended reality and deep tech.
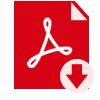