admin | 15/09/2020
In industry, Advanced Analytics has found the perfect context for forging synergies, where the production process catalyzes all phases of the value chain and technologies optimize operations. Its implementation, making it possible to predict events and identify useful patterns of behavior to define management approaches and take strategic decisions, has been gradually catching on in the business fabric, leading to the creation of what is known as Industry 4.0.
Statistical and mathematical techniques have been used in industrial processes since their inception. For example, when designing products, numerical simulation methods have been employed in software packages since the 1970s, and statistical quality control techniques have been in use since 1924. In this sector, analytics has found the perfect context for forging synergies, where production catalyzes each of its phases and technologies optimize operations. The digital transformation has entailed a qualitative and quantitative step forward in the collection and processing of data, leading to the creation of “Advanced Analytics”; its fostering of storage capacities and multiple analysis and processing options have subsequently become a core pillar of Industry 4.0.
David Carvajal (Director of Data & Analytics on the product line) and Jorge Torres (Senior Manager) from Minsait’s Industry division have taken some time to explain how its implementation is structured around the generalization of descriptive analytics and that it has been developed hand-in-hand with process optimization, the use of Artificial Intelligence and Machine Learning, and the use of advanced algorithms. “The new trends in industry, aimed at automation and the use of data in processes and production technologies, which include the use of a wide range of devices, require a strong and scalable analytical architecture that takes into account all the phases of the data life cycle,” explain the experts. These phases encompass from the collection and storage of mass volumes of data to real-time processing by processing tools. Finally, the operation layer must be enabled to consume and harness the insights obtained as part of these analytics and include them in activities.
Roll out of Advanced Analytics
Emerging technologies, the main advocates of this analysis, are Big Data and Artificial Intelligence techniques—the gray matter of this new industry. The first “fully forms part of the management and analysis of major amounts of data that could not be processed conventionally, and that enhances production while correcting errors,” explain the experts from Minsait. The same occurs with risk management: the collection of data against this backdrop “makes it possible to see beyond these processes, meaning that preventive measures can be applied.” Furthermore, this data makes it possible to align with the needs of customers, their consumption habits, and the parameters of quality and demand, making it possible to considerably improve the customer experience in addition to optimizing processes.
Artificial intelligence can be used to better understand business processes. “We have applied automatic learning methods that make it possible to predict patterns that help to optimize product quality and reduce costs,” they assert. What’s more, the technologies complement and enhance one another. “The capacity of Big Data to store and process unstructured data, such as images, makes it possible to enhance the use of Computer Vision to trace products, improving quality controls and detecting defective units.”
Macrodata also makes it possible to efficiently process information provided by sensors, images, and meteorological or logistical data, which are a major part of the industrial sector, facilitating the use of Deep Learning to scale. “As neural networks that require complex training, the horizontal scalability and processing capacity provided by Big Data technologies are essential,” in addition to Artificial Intelligence as the main basis for the algorithms used. This creates an entire universe of data that would be impossible for the human mind to process, but that is of huge value to real-time and long-term decisions made in the industrial sector.
Current scenario
The capacity of Big Data to store and process unstructured data, such as images, makes it possible to enhance the use of Computer Vision to trace products.
The implementation of Advanced Analytics in the business fabric is patchy, even relying on the area in question. “Generally speaking, it could be said that industry is one of the most advanced sectors when it comes to capturing structured data and applying this data, in particular in terms of operations and production, where automation is widespread,” assert our experts from Minsait. “Even when we had large amounts of data, there was a time at which the challenge was accessing it in a productive way. Now, having achieved this type of access, we are improving the way in which this data is harnessed, even including it in product/service proposals.”
Complex algorithms and analytics in general can be used in any sector, although the chosen method will have more or less potential depending on the activity in question. “On assembly lines, Artificial Intelligence applications have a huge impact, whereas processing companies (chemical, certain consumption firms, etc.), where product quality depends on the performance of key parameters during the process, gain more from Machine Learning models,” they explain; “Furthermore, companies that work with multi-product lines benefit from employing optimization algorithms to resolve issues they come up against when it comes to planning and scheduling production.”
For a company to be able to implement this technology as part of its activities, it must “have democratized its data, with employees having widespread, instant access to the information they require to reach the decisions pertinent to their role.” In this connection, training all members of the team so that they know how to handle this information is essential. “Automating all processes that form part of the data life cycle is also key so that it is possible to extract knowledge in a simple and transparent way across all levels,” in addition to a cultural change that places information at the core of corporate decision making. “Following a strategic reflection process, a vision should be formed about transforming the company into a data driven company.” Arriving at this vision requires a transformation that should encompass people and the way they work, providing them with new methods (Agile, DevOps, Crisp DM), skills (data talent) and tools (Data Lake, Data Centric architecture).”
This cultural transformation should be structured around a collaborative approach that guarantees the implementation of an analytical culture, Analytics First, across all levels of the organization, with a robust mechanism for setting priorities, implementation, analysis, and monitoring of the outcome of practical cases aimed at directly overcoming the challenges of the business. However, which guidelines will shape the future of Advanced Analytics? In the short-term, the trends seem to suggest that work will continue with automation and the use of Machine Learning for the purposes of preventive maintenance, in addition to the use of computer vision. From a disruptive perspective, processes will evolve towards Smart Manufacturing, with end-to-end monitoring via sensors that optimize processes, reduce costs, and improve product quality. “We are seeing the era of collaboration between progressively more autonomous robots and humans, learning from them and offering a range of capacities that are well beyond those currently used in manufacturing,” they conclude.
Analytical fields and applications
The evolution of Advanced Analytics is unstoppable and forms a core part of Industry 4.0. Our experts from Minsait have broken down the different uses of this technology in the sector:
DESCRIPTIVE
This is critical to the areas of production and support and implemented using real-time data analysis and collection techniques, with solutions that facilitate monitoring. When put to use, it is possible to search for and identify the main causes of poor performance, quality, or malfunctions, in addition to taking remedial action. The deviations identified are converted into alarms, which digitally warn those responsible for the operation.
This technique, which can be adapted to any sector or activity, makes it possible to react and prevent issues ranging from serious safety incidents to possible delays in the delivery of products and services.
PREDICTIVE
This is mainly employed in manufacturing processes and consists of analyzing data as soon as possible after it is collected (Edge Analytics). From the main predictive models, worth note are linear or logistic regression, Random Forest, decision trees, and neural networks. Each of these models has a specific use and responds to a specific question or uses a specific series of data, with the shared objective of predicting future results based on historic data.
Modeling provides results in the form of predictions represented by the degree of likelihood of the “target” variable, based on the estimate of a series of input variables. The greater the number of predictive indexes and the greater the depth of research, the higher the complexity of the analysis.
These models, as they delve into the occurrence of events, are perfect for avoiding unusual situations. The most obvious case is its use as part of maintenance. Once trained, they are capable of generating notices before malfunctions occur, with sufficient advance warning to reduce the consequences in two different ways: firstly, reducing the seriousness of the malfunction, by predicting it before the degradation mechanism causes more serious and more costly breakages; and secondly, reducing the impact in terms of lost production, as it is possible to plan repairs in advance, getting the material ready in advance and ensuring the intervention is the most time-effective possible.
PRESCRIPTIVE
This can be applied to any industrial process that needs to be improved. Its natural remit is process optimization, although it can also be applied to the field of Computer Vision applied to robotics, as it provides a solution during decisive phases such as quality control or detecting defective products. Due to the processing and application of Machine Learning algorithms to process these images intelligently, the system can take automated decisions to reverse errors and dispose of products while complying with the parameters used to configure the system.
There is currently a line of research dedicated to this field that is based on the use of Bayesian inference, a method of reasoning that adds sample data (or experimental data) to preliminary information using Bayes’ theorem. This improves decision making and the automation of actions, two outputs that are inherent to the production process, especially in terms of large-scale industry.
As part of prescriptive analytics, an additional category could be included: “OPTIMIZATION,” given its specific application to the supply chain. As our experts from Minsait explain, applying optimization techniques to business processes involved in supply chain management (chain definition, definition of inventory policies, production planning, production of programming, etc.) has a huge impact on the business.
Article collaborators:
Jorge Torres is Senior Manager at Minsait. Jorge heads up manufacturing product and project development in the Industry 4.0 division. He has 17 years of international experience in business consultancy, industry, and research. He has worked for 7 years as Project and Engineering Manager at industrial firms (automotive, energy, and engineering sectors). He has led international transformation products with a focus on operating excellence and product industrialization and design projects. He is responsible for developing the Onesait solutions that apply advanced analytics to manufacturing (Prediction and Scheduler) and serves as an expert in advanced analytics projects applied to industry. Jorge holds a degree in Industrial Engineering from the Polytechnic University of Madrid and an Executive MBA from the Industrial Organization School
David Carvajal is Director of the Data & Analytics department on the product line at Minsait. He has 20 years of experience in data technology at major firms and more than 10 years of management experience in areas relating to Big Data, Advanced Analytics, Digital Transformation, and Data Governance; he is responsible for creating, leading, transforming, and evolving the data area, covering the value chain from end to end, from governance to prescriptive analytics, and helping customers to adapt their businesses and processes to the data driven model. He holds a degree in Computer Engineering and Law, an EMBA from the Business School-IDE, and frequently collaborates with magazines, delivers conferences and seminars, and teaches at business schools, including the IE Business School.
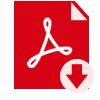